Everyone knows that “correlation does not equal causation.” If A is correlated with B, that does not mean “A causes B.” Maybe, actually, it’s B that causes A, or some third variable C causes both A and B.
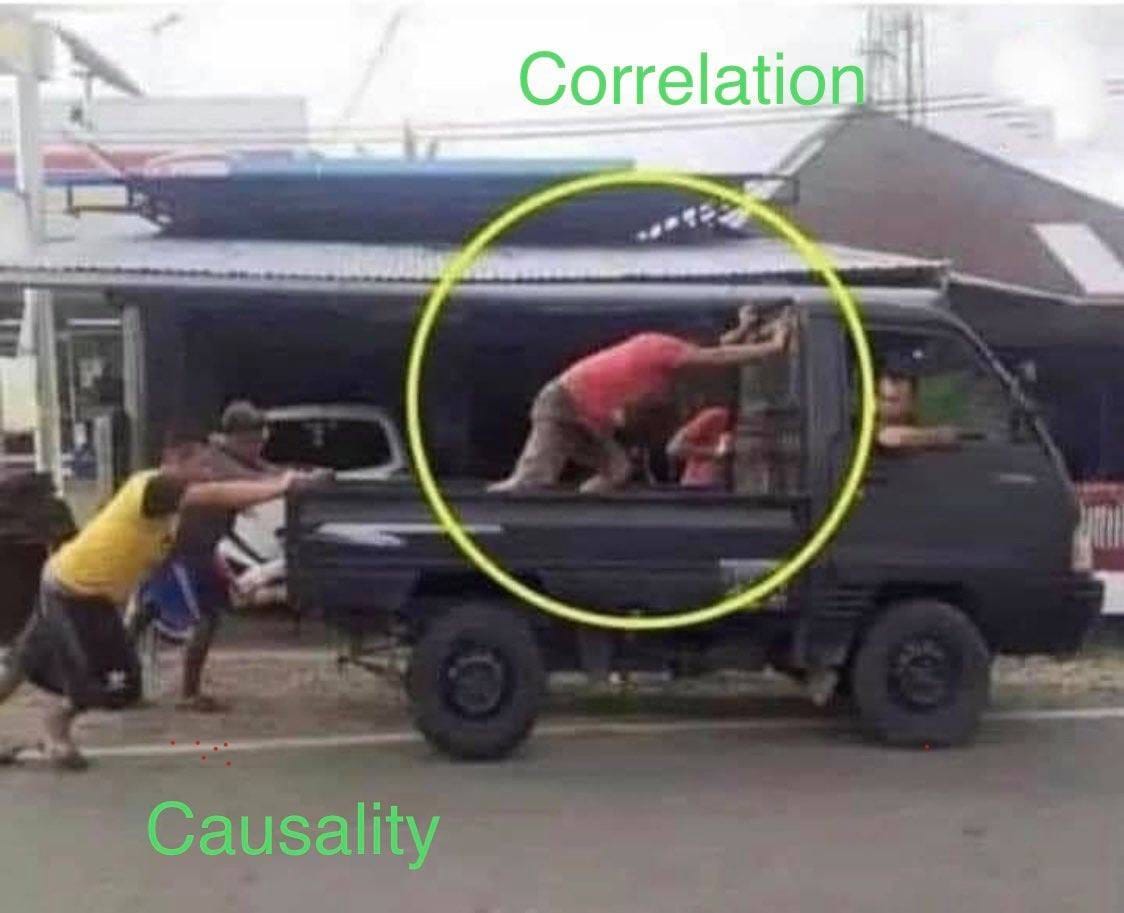
Many folks, however, seem to interpret correlation as a percentage. That is, alas, wrong. Correlation is not a percentage. If you interpret the correlation coefficient as a percentage, you can go seriously astray.
Here’s an example. In a fascinating EconTalk episode, Russ Roberts spoke to Marc Andreessen about the promise of AI. Marc Andreessen commented on the link between intelligence and positive life outcomes (such as health or educational attainment):1
Look, when IQ is linked causatively to things […] it's like a 0.4, 0.5 correlation. […] [T]here's two ways to look at that, which is, 'Wow, that's half or less than half of the cause,' which is true.
But, the other way you can look at it is in the social sciences, those are monster numbers. […] [A]lmost nothing is 40% causative on anything out of a very small number of exceptions.
To be concrete, suppose you measure a correlation of r = 0.4 between IQ and income. Then, IQ explains not 40% of the variation in income but
40% vs 16% is a 2.5x difference!
(I don’t mean to single out Marc Andreessen for an off-the-cuff comment; I have seen correlation interpreted as a percentage in academic economics seminars, Substack posts, podcast episodes, business meetings, etc. Andreessen's comment is simply a recent example I could find documented.)
Why do we need to square the correlation coefficient? The reason is that once you do that, you get the coefficient of determination (a.k.a. R-squared). It’s the R-squared that tells you what percentage of the variation in income is predicted by IQ, not the correlation coefficient.
So, don’t interpret the correlation coefficient as a percentage. If you want to quantify things like “percent of variation explained”, use the coefficient of determination.
I am always way more interested in the r squared value for this reason