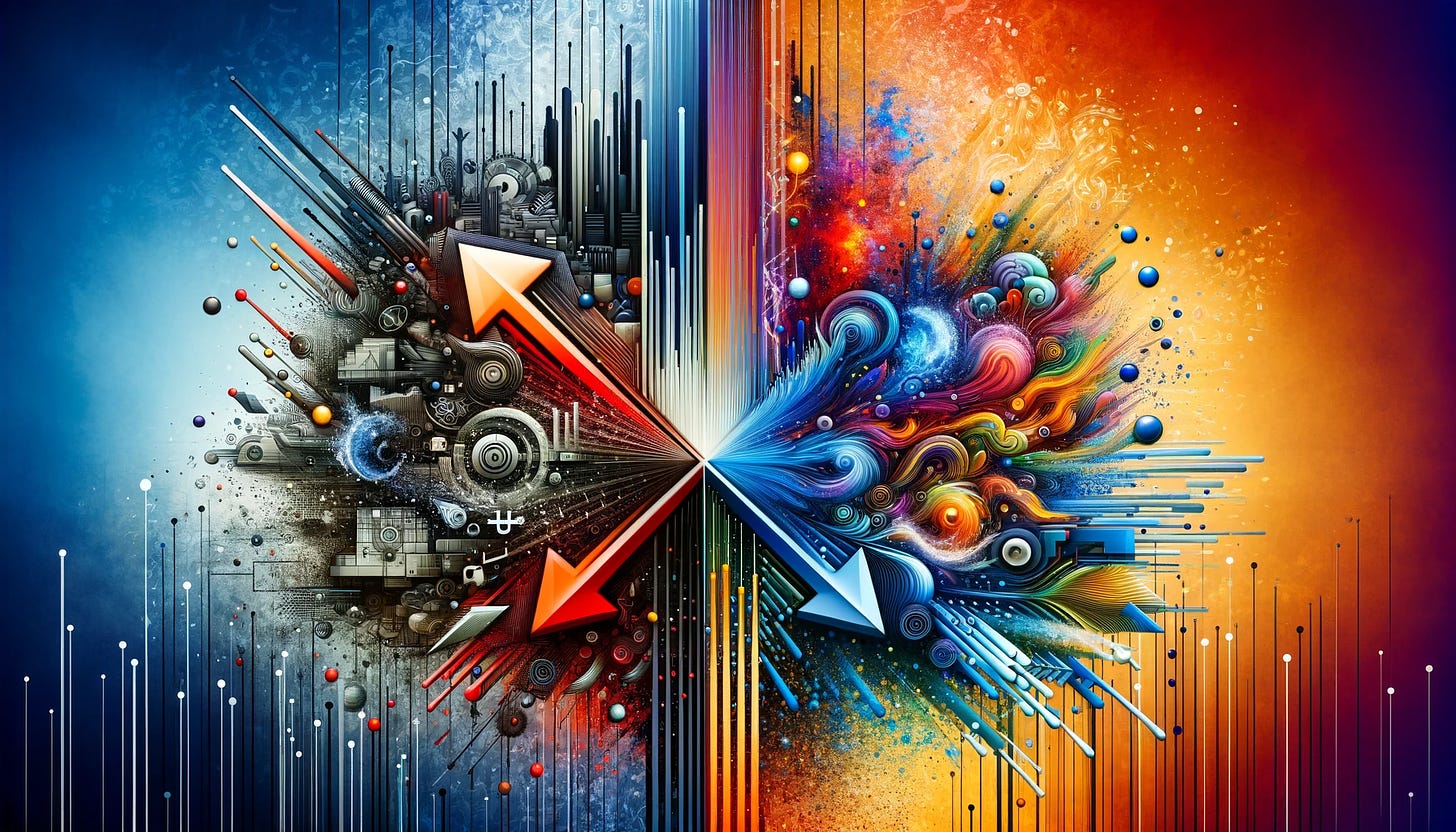
Isaac Asimov once said that the most exciting phrase in science is not “Eureka,” but “That’s funny.”
This post is about how a that’s-funny moment led to an online experiment, an alert about viewing adult content in the office, and a peer-reviewed publication in The Economic Journal.
This paper is titled “Expectation Formation with Correlated Variables.” It’s coauthored with Simin He, an awesome academic and just an overall great human. The article studies how people respond to new information when forming expectations. This question is important for everyday choices, from investors reacting to stock market changes to shoppers dealing with rising prices. (For more context, see my previous Substack.)
We found that with more information, you get more underreaction. Here’s how we got there.
“That’s funny”
Back in 2017, I was reading a new working paper by Augustin Landier, Yueran Ma, and David Thesmar.1 Landier, Ma, and Thesmar came up with an elegant forecasting experiment. In their experiment, you had to predict the next two values of a time series:
If you predict accurately, you get points—which are later converted to real money. That provides you with an incentive to do a good job.
Landier, Ma, and Thesmar found that people tend to overreact in this experiment. That is, if people see past values going up, they usually over-extrapolate.
At first, I thought: “What a cool experiment.”
It really is a cool experiment. However, Landier, Ma, and Thesmar wanted to figure out whether people under- or overreact. But in an experiment like theirs, why would you ever observe underreaction? We usually think that underreaction occurs because people don’t pay enough attention or face complex information.2 In a single-variable prediction task, though, you have all the information right in front of your nose. There’s no obvious reason for underreaction.
That’s funny, right?
Cagematch of two treatments
This insight was the impetus for the project: How can we design a clean, elegant experiment that gives underreaction a real chance?
There was only one problem: I had never run an experiment. Enter Simin, a former PhD classmate and an expert in experimental economics, who eagerly joined the project. The rest, as they say, is history.
Well, not quite. The next step was to figure out the right experimental design. After much brainstorming, Simin and I settled on the following idea. How about comparing expectations in a single-variable forecasting task—such as the one in Landier, Ma, and Thesmar—to a setting with two variables? You would still need to predict a single variable. However, instead of only looking at one variable, you would now need to consider two. We had to ensure that the comparison of the two treatments was apples-to-apples. I’ll spare you the mathematical details, but it took us many pages of time-series algebra to get that right.
This design captures some key elements of how the real world works. For example, an investor analyzing a company doesn’t rely just on its past stock price—they will also scrutinize the company’s financial accounts, market trends, and macroeconomic indicators. A doctor diagnosing a patient will take symptoms, medical history, and lab results into account to come to a conclusion. Real-world decisions are hardly ever based on a single piece of data. However, none of that complexity was present in the original experiment by Landier, Ma, and Thesmar.
In our experiment, we had two treatments. Baseline was just a replication of Landier, Ma, and Thesmar. In Correlated, however, you were shown two variables and asked to predict one of them:
We conducted this experiment on Amazon’s Mechanical Turk in 2019 July, roughly a year and a half after Simin and I joined forces.
What did we find?
Two key results. First, people are much less accurate when faced with a correlated variable. The situation got worse whenever the extra variable contained more information.
Second, people underreact to new information in Correlated. One way to see this is by checking if the forecasts are well-calibrated:
If people are well-calibrated, their forecasts should lie on the 45-degree line. In Baseline, people turned out to be fairly well-calibrated. However, in Correlated, their forecasts were compressed towards the simple default of predicting 100. That’s a strong hint that people ignore information in Correlated.
Formal statistical tests confirmed this intuition: While people exhibit overreaction in Baseline, their response flips to underreaction in Correlated.
Back to the drawing board
I know what you’re thinking now: “Didn’t you mention something about, uhm, adult content in the office? I’m only reading to find out what’s the deal with that.”
OK, about that. After finishing the experiment and writing up the results, we submitted our paper to The Economic Journal. When we received referee reports, a key theme was “Maybe the participants were just confused.” That’s a legitimate concern. Maybe folks did not know what to do with the extra information in Correlated, and they ended up ignoring the new variable.
To address this concern, we designed two brand-new treatments, which we ran in 2022.
In the first of these new experiments, we explicitly told people the following:
Future values of “Variable A” are positively correlated with past values of both “Variable A” and “Variable B”. That means that when “Variable A” or “Variable B” is larger in the current period, “Variable A” tends to be larger in the next period. When making predictions, you should therefore try to use information contained in past values of both “Variable A” and “Variable B.”
We also tested participants’ understanding of the experiment in a quiz; they were only allowed to proceed to the prediction task after successfully answering all of the test questions.
In the second new experiment, on top of all the above, we also provided participants with the exact formula for the data-generating process.
Implementing these changes in an experiment meant rewriting a bunch of code. At that point, I had already transitioned away from academia. Simin and I were collaborating with a web development firm to program the experiment. This company, for reasons unknown, opted for some highly unconventional domain names. When I attempted to review the experiment on my work laptop, I was greeted not with the experiment, but by an alert that the “website potentially contains adult content,” and that the website URL would be reported to my company’s IT department.
Not my brightest moment.
Anyway, thanks to the Editor and referees at The Economic Journal, not only did we run these new treatments, but we also fully replicated our initial findings. Everything went through, so I feel we have strong data behind us when I say that correlated information leads to less accurate forecasts and more underreaction. More info, more problems.
Lessons learned
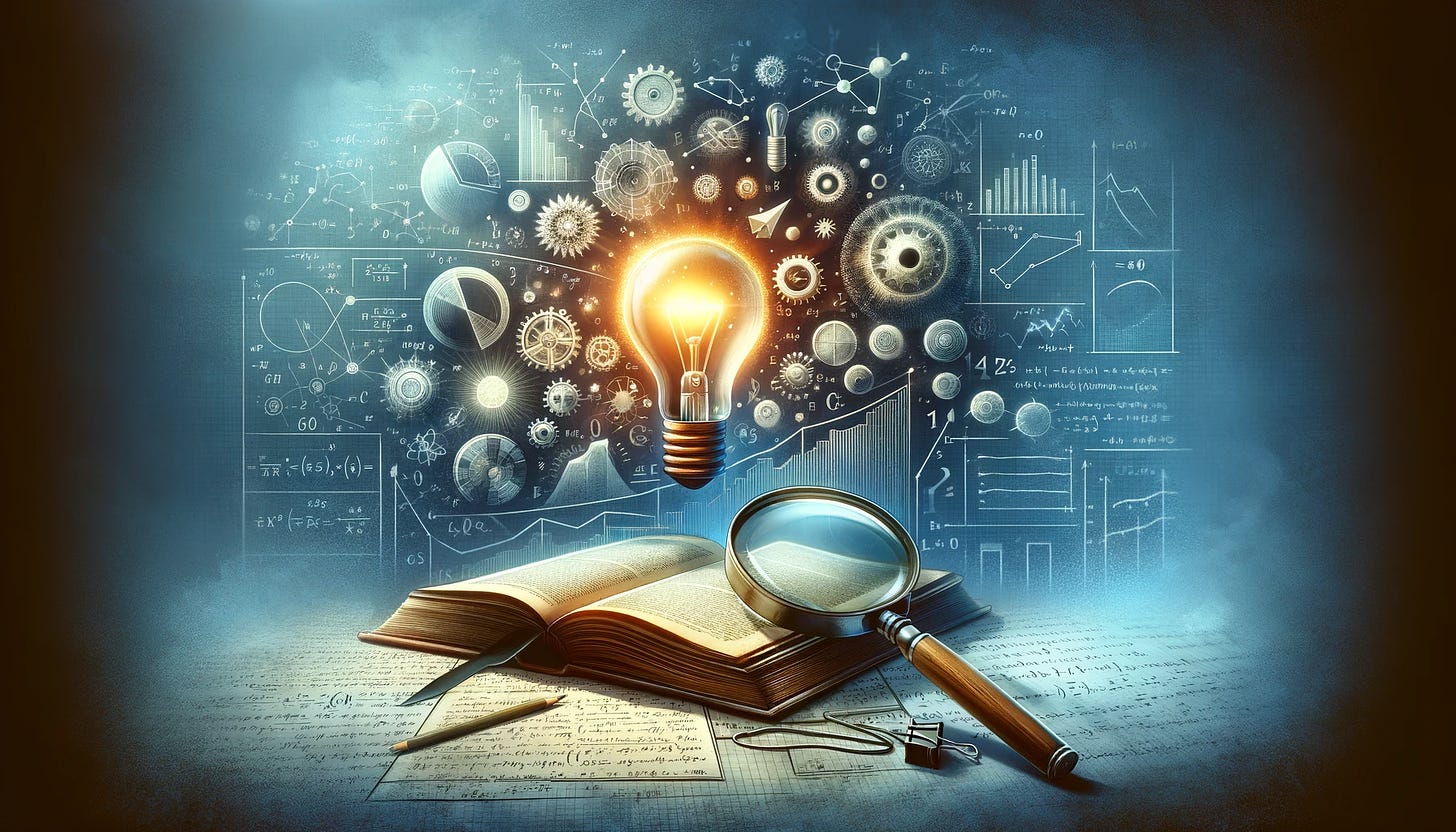
OK, so what have we learned in the end?
For me, this project was a tremendous growth opportunity. I had no prior experience in experimental economics. Designing an experiment, collecting data, and analyzing it—that was all new. I can only thank Simin for being a fantastic teacher and collaborator.
We pre-registered the experiment and our analysis plan. That required a lot of effort. I’m not sure if this effort was appreciated as much as it maybe should have been. After all, it’s pretty easy to p-hack, or do post-hoc theorizing about what caused what. In the hierarchy of empirical evidence, randomized trials are superior to observational studies, and preregistered randomized trials are superior to non-preregistered randomized trials. Unfortunately, in practice, this hierarchy is often overlooked.
Our key research takeaway—correlated information leads to underreaction—seems robust. We have literally replicated our main finding. That said, we don’t have all the answers. Why does this underreaction happen? It's intuitive that “two variables are more complex than one,” but what are the exact cognitive and psychological drivers? Just like Landier, Ma, and Thesmar, we ran our experiment on Mechanical Turk. Would these results hold in different environments, for example, with professional forecasters? These are all fruitful avenues for future research.
All in all, it was a fun, tough, and sometimes mildly embarrassing ride. As it apparently so often does, it all started with “That’s funny.” My hope is that our work sparks a similar that’s-funny moment in someone else, continuing the cycle of discovery.
A much-revised version of the paper was recently published—together with Hassan Afrouzi and Spencer Kwon—in the Quarterly Journal of Economics.
See, for example, models of rational inattention or noisy information.